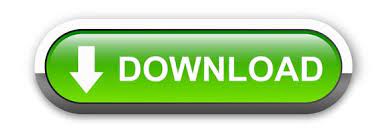
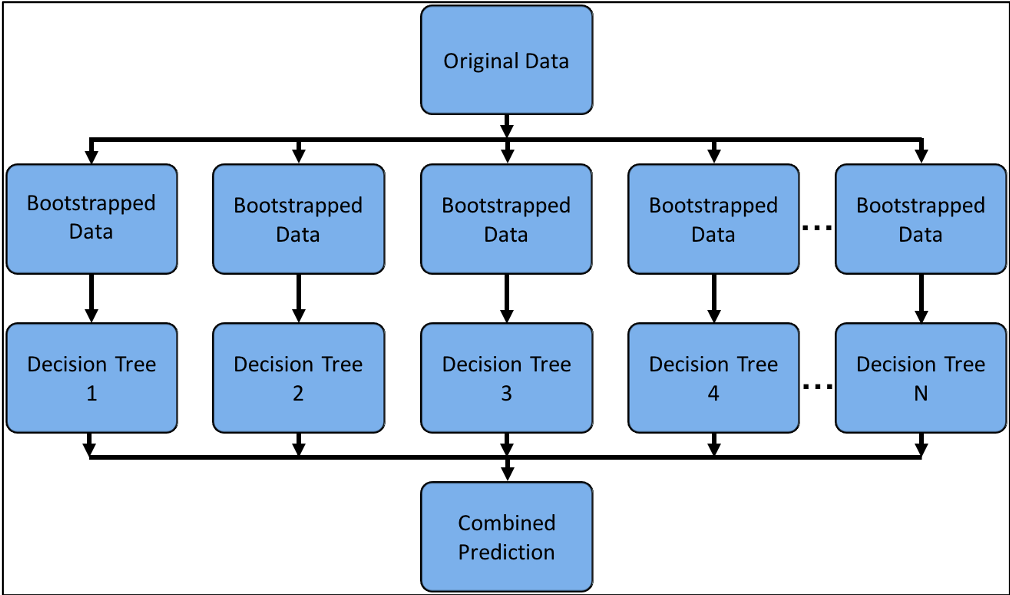
The goal is to select the feature that results in the lowest entropy after the split, as this will result in the most pure subsets of data. The default value is “gini” which is another impurity measure used in decision tree algorithm.Įntropy and information gain are important concepts in decision tree modeling that allow us to select the best feature to split the data on at each level of the tree. The tree.DecisionTreeClassifier class has a criterion parameter that can be set to “entropy” or “gini” to specify the impurity measure. In Python, the entropy and information gain can be easily calculated using the scikit-learn library’s tree module. The feature with the highest information gain is selected as the decision rule for the current level of the tree. It is calculated as the difference between the entropy before the split and the weighted average of the entropy after the split. Information gain is the reduction in entropy that results from a feature split. In decision trees, the goal is to select the feature that results in the lowest entropy after the split, as this will result in the most pure subsets of data. On the other hand, the entropy of a set of data with an equal number of instances belonging to each class is the highest. The entropy of a pure set of data, where all the instances belong to the same class, is zero. It is defined as the sum of each class’s probability multiplied by the probability’s logarithm. They are used to determine the best feature to split the data on at each level of the tree.Įntropy is a measure of the impurity or disorder of a set of data. Entropy and Information GainĮntropy and information gain are key concepts in decision tree modeling.

By the end of this tutorial, you will have a solid understanding of decision trees and be able to use them in your machine-learning projects. This tutorial will cover the steps of creating a decision tree model, including data preprocessing, model building, evaluation, and visualization. Additionally, decision trees are not sensitive to missing data or outliers, and can handle both categorical and numerical features. The tree structure of the model allows for easy visualization of the decision rules and outcomes, making it easy to understand the reasoning behind the predictions. One of the key advantages of decision trees is their interpretability. The final outcome, or the predicted class or value, is determined by the leaf node that the data falls into. The decision rules are determined by the features of the data and are represented by the branches of the tree. They work by recursively partitioning the data into smaller subsets, called leaf nodes, based on a set of decision rules.
#VISUALIZE DECISION TREE PYTHON WITH GRAPHVIZ HOW TO#
This tutorial will cover the basics of decision trees, including the concepts of entropy and information gain, and how to implement them in Python using the scikit-learn library. They are easy to understand and interpret, making them a popular choice for both beginners and experienced practitioners. Decision trees are one of the most widely used and versatile machine learning algorithms.
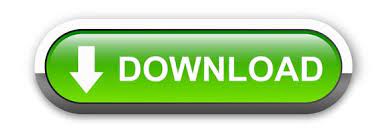